Our main research activities
The classic regularisation theory is based on the assumption that the searched-for quantity is stationary during the data acquisition. However, in many applications from medicine to non-destructive testing, this assumption is not fulfilled, for example when investigating cross-scale structural changes of materials under load experiments or moving liquid fronts in porous media.
Applying the standard techniques from the literature provides a solution with low spatial or temporal resolution and introduces motion artefacts which impede a reliable diagnosis. Therefore, our research strives after developing a comprehensive regularization theory for dynamic inverse problems, new mathematical models with a specific treatment of the dynamics and new inversion schemes.
Developing new imaging modalities and new fields of applications necessitates novel approaches in mathematical modelling, data processing and image reconstruction. A special focus of our group lies in this respect on computerized tomography and microscopy.
The relentless progress in imaging technology and the exploitation of new fields of application require new mathematical models and numerical solution methods. The special focus of our group lies on classical modalities like computerized tomography or magnetic resonance imaging as well as on novel tomographic methods like magnetic particle imaging.
In order to extract features and properties of the studied medium, the reconstruction results are typically subject to further processing steps. Since an ever-increasing amount of data arises in modern applications, special algorithms are required to extract the desired information in an efficient and stable way. In this context, it is desirable to obtain this information directly from the data, i.e. in the form of a feature reconstruction.
Classical solution schemes for inverse problems are based on mathematical models which describe the link between data and searched-for quantity. For many classes of such model-based reconstruction methods, their convergence properties are well understood.
In novel, data-driven reconstruction methods, the sought-for quantity is determined by the evaluation of a neural network whose parameters have previously been trained on suitable test data. Thus, redundancies, structures and features in the test data can be identified and used for the solution step without explicit modeling.
A major difficulty of purely data-driven approaches is to establish a control over the output. Therefore, we investigate in the project iDeLIVER the combination of model-based and data-driven methods in order to enable a high acceleration of the reconstruction step without compromising the e.g. diagnostic validity.
Current research projects and cooperations
Project coordinator: Prof. Dr. Bernadette Hahn-Rigaud (Prof. Dr. Holger Steeb)
Project period: 2023-2025
Funding institution: SimTech EXC 2075, German Research Foundation (DFG)
Project description:
This project addresses challenges arising in computerized tomography (CT) of rheological experiments which are due to the dynamic behaviour of the material under study. Since the CT-data acquisition takes a considerable amount of time, only a limited amount of data can be recorded for each state of the object resulting in severe artefacts in the reconstructed images. We therefore strive to develop appropriate reconstruction algorithms by combining data-driven techniques with a suited physical process. Ignoring the temporal correlation between the different states of the material under study in rheological experiments leads to reconstructed images with severe undersampling and/or motion artefacts. This can be encountered for by including suitable a priori information, for instance about the static structure of the specimen, which can be recorded via a high-resolution scan before the dynamic experiment starts. To this end, we focus in a first step on the characterization and estimation of the motion from large datasets guided by inconsistency detections in the data and the optical flow equation. Our subsequent goal is then to combine this derived information with reconstruction approaches, such as RESESOP or learned primal dual, able to handle imperfect models. Finally, we propose to enhance the quality of reconstruction and learning by considering the propagation of singularities, understood by microlocal analysis, and differentiating between features and artifacts.
Research Assistant (University of Stuttgart):
MSc. Mathias Feinler
PN 1-10: Combining imaging, physics and analysis for rheological experiments
Project period: 2021-2025
Funding institution: Sino-German Center for Research Promotion (Chinesisch-Deutsches Zentrum für Wissenschaftsförderung (CDZ))
Project description:
In this mobility programm, a multidisciplinary team of Chinese and German researchers in the field of inverse problems will exchange and pursue knowledge and ideas on the modelling, mathematical analyzing, algorithm developing, implementing and validating from the classic to modern perspectives.
Involved research and application partners within the project:
Principal Investigators from China:
- Coordinator: Prof. Ming Jiang, Peking University
- Prof. Jin Cheng, Fudan University
- Prof. Shuai Lu, Fudan University
- Prof. Xiaoqun Zhan, Shanghai Jiao Tong University
Principal Investigators from Germany:
- Coordinator: Prof. Peter Maass, University of Bremen
- Prof. Bernadette Hahn-Rigaud, University of Stuttgart
- Prof. Alfred K. Louis, Saarland University
We currently hold external cooperations ie. with the following institutions:
- University of the Saarland, Germany
- University of Bremen, Germany
- Julius-Maximilians-University Würzburg, Germany
- Universitätsklinikum Würzburg, Germany
- Friedrich-Alexander-Universität Erlangen-Nürnberg, Germany
- Fraunhofer Institute IIS, Erlangen, Germany
- Georg-August-University, Göttingen, Germany
- Tufts University, USA
- Peking University, China
Completed research projects and cooperations
Project ccordinator: Prof. Dr. Bernadette Hahn-Rigaud
Project period: 2020-2023
Funding institution: Federal Ministry of Education and Research (BMBF)
Project description:
The project examines the use and further development of machine learning methods for MR image reconstruction and for the classification of liver lesions.
Based on a comparison model and data-driven image reconstruction methods, these are to be systematically linked in order to enable high acceleration without sacrificing diagnostic value. In addition to the design of suitable networks, research should also be carried out to determine whether metadata (e.g. age of the patient) can be incorporated into the reconstruction. Furthermore, suitable classification algorithms on an image basis are to be developed and the potential of direct classification on the raw data is to be explored. In the long term, intelligent MR diagnostics can significantly increase the efficiency of use of MR hardware, guarantee better patient care and set new impulses in medical technology.
Involved research and application partners within the project:
- Prof. Dr. Bernadette Hahn-Rigaud, Chair of Optimization and inverse Problems, University of Stuttgart
- Prof. Dr. Alfio Borzì, Chair of Mathematics IX, Scientific Computing, JMU Würzburg
- Prof. Dr. Andreas Maier, Pattern Recognition Lab, FAU Erlangen-Nürnberg
- Prof. Dr. Herbert Köstler und Prof. Dr. Tobias Wech, Experimental Radiology, Universitätsklinikum Würzburg
- Prof. Dr. Thorsten Bley, Department of Diagnostic and Interventional Radiology, Universitätsklinikum Würzburg
- Dr. Moritz Berger, Siemens Healthcare GmbH, Erlangen
- Prof. Dr. Karsten König und Dr. Andreas Schindele, JenLab GmbH, Berlin
Research Assistant (University of Stuttgart):
MSc. Mathias Feinler
Project period: 2020-2023
Funding institution: German Research Foundation (DFG)
Project description:
The project deals with three dynamic aspects in MPI (concentration dynamic, magnetic field dynamic and particle magnetizing dynamic) which lead to a numerous number of dynamic inverse problems. The goal is to develop reconstruction methods which explicitly include the dynamic behaviour.
Involved research partners within the project:
- Prof. Dr. Bernadette Hahn-Rigaud, University of Stuttgart
- Dr. Tobias Kluth, Zentrum für Technomathematik, University of Bremen
Research Assistant (University of Stuttgart):
MSc. Marius Nitzsche
Project period: 2017-2021
Funding institution: German Research Foundation (DFG)
Project description:
This project deals with the development of a regularization theory for dynamic imaging. The additional time dimension alters the degree of ill-posedness, the spatial resolution or leads to limited data issues.
These aspects will be analyzed and suitable regularization procedures will be developed.
Research Assistant (University of Stuttgart):
MSc. Melina-Loren Kienle Garrido
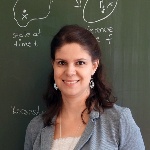
Bernadette Hahn-Rigaud
Prof. Dr.Chairholder OIP
- Profile page
- +49 711 685 61605
- Write e-mail
- Prof. Bernadette Hahn-Rigaud